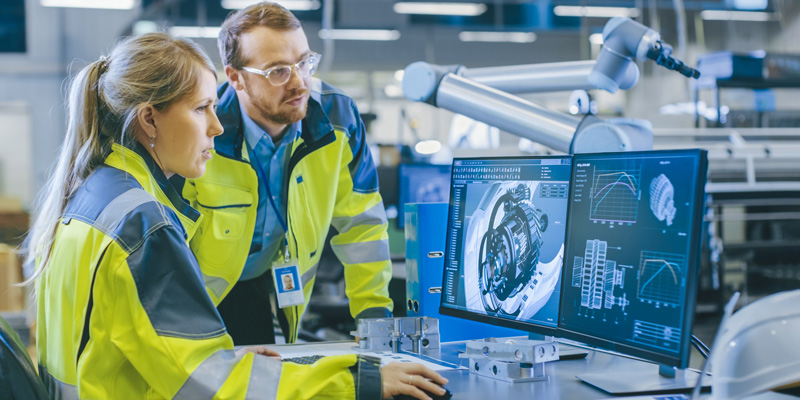
Projecting jobs growth from capital investment
Clinical Associate Professor of Business Economics, Indiana University Kelley School of Business
Data Analyst, Indiana Business Research Center, Indiana University Kelley School of Business
Talent is the new currency of regional economic prosperity. A region becomes more competitive if it can more precisely predict workforce needs and more proactively develop the talent that employers will hire.
When faced with the potential for new business investment, one of the most important questions for economic developers is how many jobs will be created because of the investment. The answer to this question has wide-ranging implications, from the incentives the state will offer the investing company to the number of new degree-earners local universities should graduate in the next five to ten years. Talent is the new currency of regional economic prosperity. A region becomes more competitive if it can more precisely predict workforce needs and more proactively develop the talent that employers will hire.
State economic development organizations rely on the companies themselves to provide job figures, as well as economic modeling to generate estimates of the jobs that will be created or retained because of the investment. This requires trust that the company is providing good-faith and accurate job estimates, as well as modeling expertise from the economic developers. The challenge for both parties is the relative lack of information and inherent uncertainty about the impacts of the investment. The availability of data determines the model that can be built. The industries that drive a regional economy and the occupational groups that fill their jobs can be very specific. Modeling is often hampered by a lack of industry and occupational detail within government data sets offered at a regional level.
Higher levels of precision help state policymakers better align business recruitment, tax incentives and infrastructure investment decisions with the capacity of a region to develop and attract the talent that local companies need.
Stronger techniques for regional workforce prediction can strengthen a state’s ability to set economic strategy, formulate policy and recruit new investment. This article offers a blueprint for predicting detailed job impacts by industry and occupation given a new capital investment. Higher levels of precision help state policymakers better align business recruitment, tax incentives and infrastructure investment decisions with the capacity of a region to develop and attract the talent that local companies need.
Motivation for the model
A company’s projection of job creation from a given investment can be highly subjective and politically motivated, especially when there is negotiation with local and state authorities for tax benefits and new public assets. Methods used to predict additional employment are not consistent from one project to another. While economic developers can use economic modeling software such as IMPLAN to make their own projections, for the most part, they take companies at their word in their projection of employment creation. There is acceptance that companies are the experts on their labor force needs.
Job growth projections provided by a company proposing a capital investment do not consider secondary economic impacts, whether in the same or in other industries. Moreover, how these impacts induce job creation or create a need for more workers with specific educational credentials is unknown. For a given capital investment in a specific industry, there is need for a model that estimates primary and secondary employment growth by occupation and the expansion in regional educational capacity required to staff these new jobs.
Embryonic framework for the model
Knowledge of training requirements by occupation enables prediction of how many more workers with certain credentials are required to fulfill a region’s expanded talent needs.
The model uses a modular estimation design to link capital investment to job growth by occupation and needed expansion in regional educational capacity. See Figure 1 for a simple graphical representation of the model components. A given capital expenditure generates GDP growth in its primary industry. Growth in the primary industry generates secondary GDP growth in other industries. GDP growth across industries requires employment growth in occupations that are inputs for those industries. Certain occupations require advanced training or a college degree and other occupations require just a high school diploma. Knowledge of training requirements by occupation enables prediction of how many more workers with certain credentials are required to fulfill a region’s expanded talent needs.
Figure 1: Formula behind the model projecting jobs growth and degree needs by industry
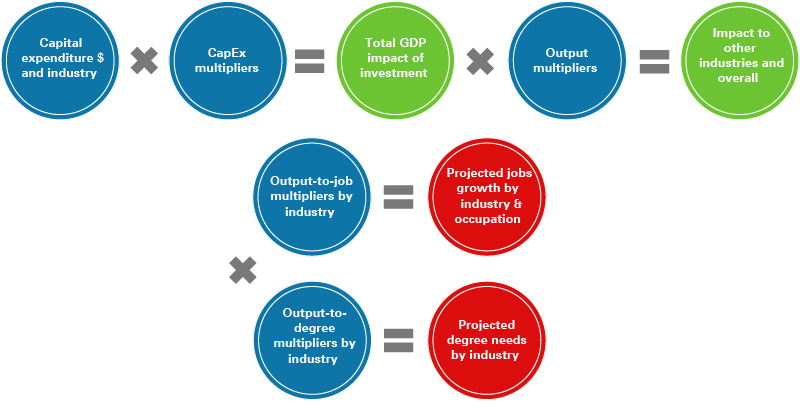
Source: IBRC
Estimation requires the sophisticated application of multipliers. The capital expenditure multiplier used to estimate primary GDP impact must account for differences in capital and labor intensity across industries. Small capital expenditures generate large additions to employment in labor-intensive industries and small additions in capital-intensive industries. Knowledge of capital and labor shares by industry are therefore important for model construction. Secondary impacts on GDP are calculated using cross-industry multipliers provided by IMPLAN software.
Conversion of GDP impact to occupational employment growth impact uses linear production functions estimated for each industry. Such functions estimate how many more full-time equivalents in each occupation are needed to produce one more dollar of GDP in a given industry. Because of its detail and completeness, use of national data allows production functions that map every specific industry to every specific occupational category. The methodology uses production functions estimated with national data to estimate impact for a single state. This is statistically consistent if the relationship between talent and output in the industries of interest are similar at the national and state levels.
Categorization of employment growth by occupation allows mapping of each new job to training requirements. Data from the U.S. Bureau of Labor Statistics identifies the standard educational attainment for each occupation. This information is used to estimate how many new recipients of certifications and degrees are needed to fill the new jobs created by the capital expenditure.
Applications of the model
Given a capital investment amount and an industry, one can project jobs growth for nearly 800 occupations (as categorized by the Standard Occupational Classification system) in 85 three-digit subsectors (as categorized by the North American Industry Classification System). Such a model is versatile not only in predicting the workforce impact of new capital investment, but also in assessing how well the current workforce meets the needs of a regional economy.
Growth in high-wage, high-demand jobs is of priority interest to policymakers. Newly emerging industries, such as electric vehicles, computer chips and biotechnology, and new specific occupations that support them, such as robotics engineers, data scientists and genetics specialists, receive special attention because states and regions know they are the highest value drivers of economic expansion. The model proposed here can be used to score how well new capital investments achieve the type of structural industry and workforce changes desired by government officials.
This methodology can also be used to develop state heatmaps for workforce development. Policymakers can determine which regions are primed for growth and investment in certain industries and occupations. Tendencies toward specialization, emergence of new industry clusters and decline of established industry clusters can be predicted for any region based upon changes in the strength of match between the industry structure of economic output and the occupational structure of the workforce. Dramatic changes in this match suggest extraordinary regional economic shifts typically driven by technology change, large investments and the exit and entry of old and new employers.
Tendencies toward specialization, emergence of new industry clusters and decline of established industry clusters can be predicted for any region based upon changes in the strength of match between the industry structure of economic output and the occupational structure of the workforce.
The model can be extended to appraise the sufficiency of local housing stock and the larger infrastructure that supports it. Predicted new employment volume by occupation can be stratified by expected income groups and then used to forecast new demand for housing at lower and upper ends of the real estate market. Just as educational and training capacity can be a bottleneck in upskilling a workforce to match elevated industry needs, constraints on affordable housing can also impede a sufficient supply of workers. Given new capital investment in a region, policymakers must adequately address how local communities will accommodate additions to their population.
Conclusion
Negotiation for and adjustment to new capital investment requires analysis of how well a regional workforce can meet new talent needs in certain industries. Data aggregated at a national level can be used to build a model that forecasts required regional employment volume for industries and occupations defined at a detail level. Workforce strength can be assessed in terms of how well it matches the individual industry structure of a region. Opportunities for public policy to fill talent gaps can be identified in a sophisticated way.
In practice, use of the model in policy planning requires acceptance of mathematical sophistication that often raises suspicion. The methodology must be explainable, forecasts should be believable and political neutrality has to be unquestionable. Translation of the model into practice is best done through regional case studies that quantitatively refine and place in context any forecasts offered by the model. This enables a shift to regional storytelling that makes workforce assessment and policy recommendations digestible for politicians and constituencies.